When artificial intelligence and machine learning transform e-commerce: a booming technological era
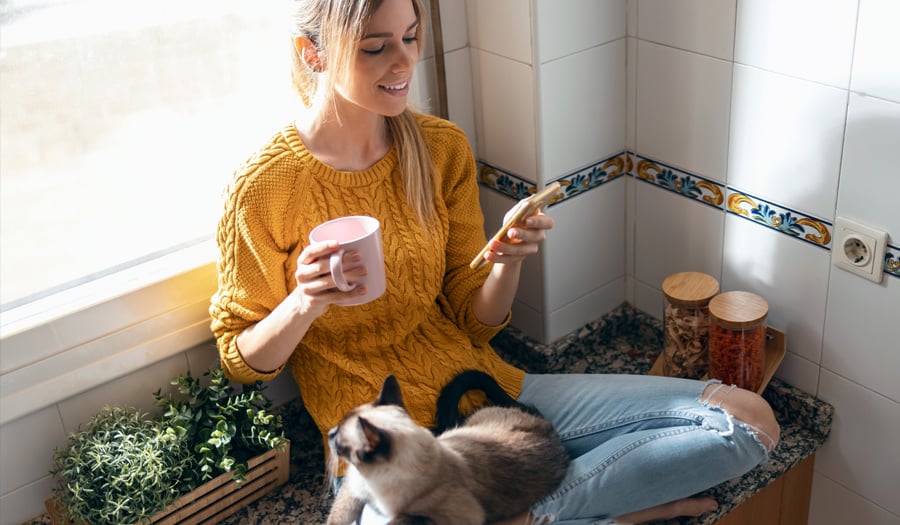
Artificial intelligence, machine learning... These 'buzzwords' have become indispensable in technological vocabulary, but how many people truly understand their profound meaning? Before delving further into this article to understand how these technologies can be leveraged for e-commerce, let's take the time to dispel the mystery surrounding them.
Artificial Intelligence (AI) is characterized by the creation of computer algorithms capable of performing tasks with 'some' intelligence. The field is very vast and includes dozens of fields of study such as distributed systems (multi-agent systems), optimization problem solving (metaheuristics), expert system construction, constraint satisfaction, and planning.
Machine learning is one of these fields of study within AI. Machine learning algorithms have the ability to learn from data and extract relevant information from it through the application of statistical rules that define a learning model. With machine learning, the developer no longer directly codes the means to solve a problem but instead codes the means to learn how to solve the problem based on data..
Machine learning generally consists of two fundamental phases: training and prediction. During the training phase, the algorithm adjusts its parameters using a data set, learning the underlying patterns and relationships present in the data. Once the model is trained, it can be deployed to make predictions or decisions on new data during the inference phase.
When you consider that an e-commerce site handles a wide range of products, attracts numerous users performing various actions (browsing, expressing interest in products, making purchases), and all of this generates a considerable amount of data... You immediately realize the significant potential of machine learning algorithms to understand visitor behavior and, as a result, directly improve the customer experience and optimize sales.
To ensure the effectiveness of these algorithms, two ingredients are necessary: high-quality data and specific usage contexts. Here are some concrete examples.
Machine learning to optimize product search
When it comes to search, machine learning can be employed in various ways:
- To optimize the automatic understanding of the semantic meaning of a query.
Let's illustrate this with a voice search. When a visitor says, "I would like a gray sofa please," the search engine, based on machine learning, can recognize that the important terms are "gray sofa" and that "gray" corresponds to a color, ignoring the rest of the sentence. Thanks to technologies derived from NLP (Natural Language Processing) or more recently through LLMs (Large Language Models), the search engine is able to semantically understand the user, even if the sentence is formulated in natural language, in a complex way.
- To suggest products in case of an unknown search expression.
In the case of an unknown search expression, it is possible to use a re-opening algorithm. This allows relevant items to be displayed, even if they have different names. For example, if a visitor enters "sombrero," the e-commerce site can suggest winter hats or straw hats for summer. This capability results from machine learning's semantic understanding that "sombrero" is similar to "hat" or "bonnet.
Another aspect of applying these algorithms is to personalize the customer journey.
Machine learning algorithms open up prospects for personalization.
This can be done notably at the product ranking level and manifests in various contexts:
- Seasonality: The ranking can vary according to the seasons. For example, if visitors search for sweaters in summer, the system will prioritize lighter articles, while in winter, it will highlight thicker and warmer sweaters.
- User preference: When a user performs product searches for men's items and enters the term "shoes," the search results can be directed towards men's shoes.
- User profile: It is possible to learn how to classify customers and define typical profiles that can then be used to personalize search engine responses or recommendation algorithms, or even to create personalized product listings on the homepage, for example.
- Typed keywords: When the user enters "jeans," the search engine understands that they are primarily looking for pants rather than denim jackets, a deduction drawn from its learning of user expectations. By adjusting rankings based on keywords, machine learning organizes products in a relevant way, incorporating notions such as best-sellers.
Personalization can also extend to other aspects, such as:
- Product listings: Suggestions can be made for popular products that complement the one the user is viewing. This feature relies on cross-sell algorithms, identifying products frequently purchased together. Additionally, it's possible to display choices made by other customers who have bought the same product, offering complementary suggestions for a cart. Another possibility would be to suggest similar items but in a higher price range (up-sell).
- Homepage: Personalized products can be offered to the user based on their history. This can be done in various ways: as an opening to introduce them to items they haven't discovered yet but might be interested in, or as a closing to suggest products they have already purchased or frequently acquire. This approach is particularly relevant in food retail, where recommending regular products enhances the shopping experience.
The mentioned use cases focus on direct improvements to the customer experience. In parallel, machine learning can also contribute to providing insights for the individuals managing the e-commerce site to improve their professional tasks.
Machine learning algorithms: powerful allies for e-merchandisers
Let's consider a scenario where a group of products appears to be poorly organized within a category. Machine learning algorithms have the ability to detect this situation and flag it. Human intervention would then be necessary to evaluate and qualify the category. In addtion, algorithms can also identify categories that lack relevance, grouping a variety of products without significant connection, and suggest dividing them into more specific subcategories.
These algorithms also provide e-merchandisers with suggestions of relevant synonyms to integrate into the back office. This feature relies on the analysis of the user's null results, allowing for fine-tuning of search results. For example, suggesting synonyms such as 'wall light' for 'light fixture', or 'nightshirt' for 'nightgown', demonstrates the ability of these technologies to anticipate and meet customer expectations.
In conclusion
The power of machine learning algorithms lies in their ability to finely assimilate user behaviors, identify trends, classify, and reveal significant correlations between products. This capability makes them indispensable tools for optimizing the overall user experience, particularly in the areas of search and recommendation. They pave the way for a more immersive and personalized user experience, helping to maximize the conversion rate on the e-commerce site.
What will the future of online search look like? It's certain that it won't be limited to keyword queries as we know them today. Recent advancements in artificial intelligence, particularly in the field of generative AI, such as Large Language Models (LLMs) capable of understanding and generating human-like text, as exemplified by ChatGPT, are paving the way for new perspectives.
These technological advancements offer opportunities to reinvent how we use search engines and could evolve towards a more direct interaction, where talking to with search engines becomes as natural as interacting with a physical store salesperson.
Tomorrow, the user experience on websites could undergo a major transformation, marking a significant evolution from our current habits.
Photo credit : ©Shutterstock
This expert opinion was featured in actu.ia, ia-news, digital solutions, media dedicated to artificial intelligence and new technologies.