The importance of product recommendation in the strategy for personalizing the user experience on e-commerce platforms
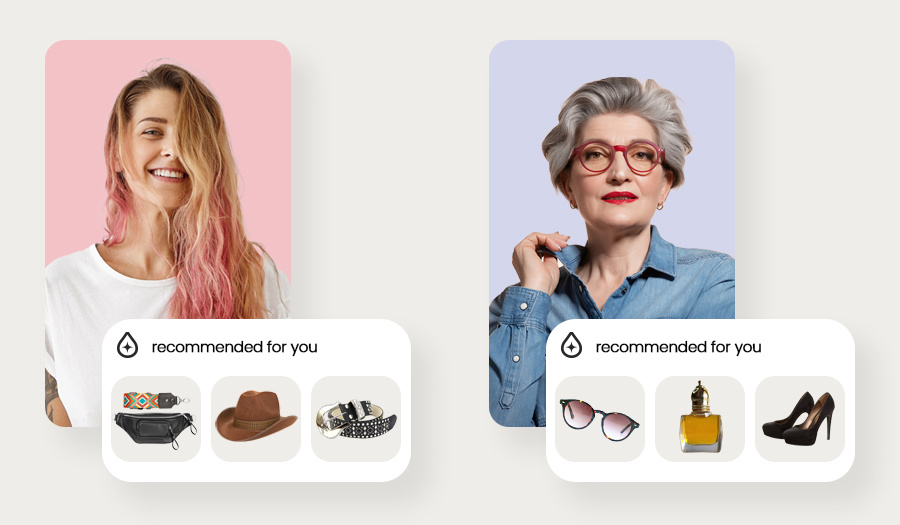
Product recommendation, an integral part of the user experience personalization strategy, allows e-retailers to demonstrate their in-depth knowledge of consumers' preferences and needs, and their ability to provide them with products that perfectly match their needs.
This ability to understand and anticipate customer expectations is based on sophisticated recommendation systems. Before exploring how these systems manage to propose relevant products to each user, it is essential to define what they are.
In English, the concept of a recommender system uses a variety of expressions, but the one that catches our attention is "recommender system". This is a holistic IT system designed to integrate and deploy different recommender modes, algorithms and use cases. This complex system combines advanced techniques to provide personalized suggestions that respond precisely to each user's expectations. These techniques include:
Content-based filtering
Content-based filtering’ distinguishes itself by focusing on the intrinsic characteristics of products. This filtering method recommends items that are similar in terms of attributes to the products the user is browsing - such as their color or material, for example.
Sensefuel has been using content-based filtering for over 5 years, via a vector stack, a key element of its solution. This technology assigns semantic vectors to each product, making it easier to calculate similarities between products. Thanks to these vectors, we can identify which products are close to each other based on their attributes.
This approach is applied to various aspects of the Sensefuel platform, including the search engine, result ranking, product selection, and many other applications. The same vectors are also used to generate personalized recommendations.
Collaborative-based filtering
Unlike content-based filtering, which focuses on product attributes, collaborative-based filtering extracts meaning from user behaviour. By following the path of each user, it is possible to deduce preferences and potentially significant correlations between different products.
So, if "User A" visits product pages in sequence and "User B" follows the same path, these products are considered related, regardless of their nature. Whether it's books or shoes, collaborative-based filtering relies on the statistical average of the behavior of a community of users to infer correlations and recommend a product when one user consults another.
The data is collected in two ways:
Explicit: the user provides information directly by rating a product or adding it to a favorites list.
Implicit: each visit to the product page, each addition to the shopping cart, and the final purchase are processed to identify consumer trends and preferences.
It is clear that the e-merchandiser has a wealth of information at his or her disposal and that algorithms play an indispensable role in recommending products. However, their effectiveness depends largely on the use cases to which they are applied.
These recommendations can be integrated into various parts of an online store (home page, product listings, search results, etc.) Let's look at some concrete examples.
- Recommendation from a product sheet
There are several possible usage scenarios for a product sheet.
The first approach is to suggest products with similar attributes, such as color and texture. For example, if the user is on a page for jeans, the e-commerce site will suggest other jeans with the same color, texture and size, but at a higher price point, to upsell.
Another usage strategy is based on purchasing behavior: suggesting items that other consumers have frequently purchased in addition to the item being viewed. This method makes it possible to recommend products that have been validated by others at the time of purchase. In the DIY sector, for example, this analysis can be used to recommend kitchen furniture from the same range or compatible accessories, without having to qualify the information beforehand.
It is also possible to use the purchase path to identify the other products that users have viewed during the same session. Users who viewed this product also viewed these other products. Although this type of recommendation is noisier, it encourages the user to discover other products.
Moreover, it is possible to combine these different approaches: analyze the products visited before a particular item was purchased to suggest possible alternatives, even if these products were not purchased. For example, if a user is considering buying a velvet jacket, it is relevant to know that buyers of this jacket have also explored other velvet jackets. Presenting these product vignettes encourages diversification of choices and opens up new avenues of exploration.
- Recommendations from the home page, based on the contents of the user's shopping basket
The e-merchandiser could consider recommending additional items based on the current contents of the user's basket and their recent purchases. For example, if the user has already added five or six items, what might be the seventh item to recommend, based on the behavior observed in the community of shoppers? By analyzing the different items in the user's cart, this method goes beyond a simple algorithmic recommendation based on a specific product sheet.
When exploring recommendation strategies on e-commerce platforms, it is important to note that Sensefuel does not limit itself to traditional methods such as collaborative-based filtering or content-based filtering. Sensefuel integrates these approaches with its core AI and advanced personalization system to improve the relevance of its recommendations.
What's more, its approach to product recommendation is not based exclusively on AI algorithms. Sensefuel also provides recommendations based on product selection. E-merchant customers can create specific assortments to promote flagship products, support promotional campaigns, and more. The recommendation algorithm not only selects the first items in the selection, but also carefully analyses users' individual preferences to choose from product assortments that best match their specific tastes. This approach takes advantage of the personalization system built into the entire Sensefuel solution, offering e-merchandisers total freedom of configuration thanks to a turnkey solution and widgets that can be integrated directly in the desired location on a page.
Sensefuel offers Plug & Play and API deployment modes, designed to allow the e-merchandiser to easily control what is displayed at any point on the site, without necessarily relying on a technical team.
In conclusion, product recommendation on e-commerce platforms is much more than a technical feature. It is a strategic tool that allows retailers to demonstrate a deep understanding of consumers' individual needs.
By integrating different approaches such as content-based filtering and collaborative-based filtering, combined with personalized selection methods, Sensefuel provides a flexible and powerful solution for optimizing the online shopping experience. This approach not only stimulates sales by offering relevant products, but also strengthens customer loyalty by ensuring a personalized and engaging shopping experience.
Discover the impact of Sensefuel on your e-commerce site!
Do you want to offer captivating shopping experiences, tailored to the sensitivities and contexts of each visitor, to convert searches into sales?
Thanks to its search, recommendation and hyper-personalisation capabilities, Sensefuel can boost your performance and meet the growing expectations of your users.